What Is Intelligent Document Processing (IDP)? How it works in 2024
Intelligent Document Processing is an advanced technology designed to streamline the way organizations handle documents, converting long-form documents into usable data and actionable insights.
While the concept may seem technical, it’s easier to grasp the benefits when we break it down and see how it impacts enterprises on a large scale.
What is Intelligent Document Processing?
Broadly, Intelligent Document Processing (IDP) refers to automation that uses advanced technologies to convert the information in documents into structured, usable data. Recently, IDP has come to include the use of AI technologies like machine learning (ML) and natural language processing (NLP) to extract, classify, and process data from various types of documents. These documents can include contracts, invoices, purchase orders, insurance claims, and more.
Traditional methods of processing such documents have relied heavily on manual labor or rudimentary automation, such as Optical Character Recognition (OCR), which can turn imagery into software-readable text, but stops there.
IDP, on the other hand, has evolved to take into account the visual structure of the information, the contextual relationships of words and phrases, and automation to structure and connect the information to other systems. Essentially, IDP takes automation a step further, transforming many types of semi-structured documents into structured information and data that businesses can use directly.
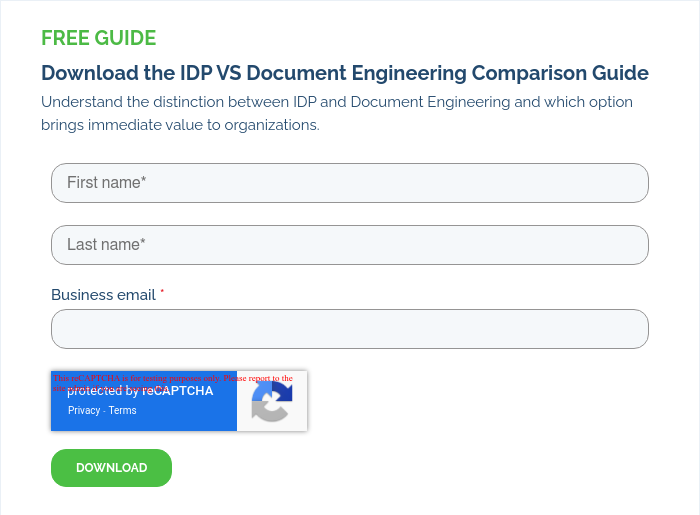
How does intelligent document processing work?
IDP combines several technologies to ensure efficient and accurate document processing:
- Document Ingestion and OCR: The first step in IDP is to ingest documents, regardless of whether they are paper-based or digital files (PDFs, images, etc.). Traditional OCR technology is often used to convert scanned images into machine-readable text. However, IDP goes beyond OCR by not only recognizing characters but also understanding their meaning in context.
- Document Classification and Data Extraction: Once the document is digitized, machine learning models classify the document type (e.g., invoice, contract, or legal document). NLP models then extract key information such as names, dates, amounts, and other relevant fields. For instance, in a commercial insurance policy document, IDP can pull out the insured party’s details, coverage limits, and policy dates with high accuracy.
- Contextual Understanding: Using NLP and deep learning models, many IDP systems are increasingly capable of understanding some elements of the context of the document, identifying relationships between different pieces of information. For instance, some IDP systems may be able to differentiate between a billing address and a shipping address based on how the information is structured within the document.
- Validation and Workflow Automation: After extracting the relevant data, some IDP systems offer the opportunity to validate it against predefined rules or cross-reference it with other data sources. Some systems offer the opportunity for a “human-in-the-loop” to manually sample and review data for accuracy. The data can then be fed into downstream systems such as ERPs or data analytics tools, where further business processes can be automated.
IDP vs. Traditional Document Processing
Traditional systems like Document Management Systems (DMS) and Optical Character Recognition (OCR) have long played roles in helping businesses store and manage large volumes of documents. However, these earlier systems come with significant limitations, particularly when it comes to handling data and performing complex tasks that require understanding, context, and decision-making.
Intelligent Document Processing (IDP) takes document management to the next level with powerful AI-driven capabilities.
Here’s a detailed comparison of traditional document processing systems and Intelligent Document Processing (IDP):
Document Storage
Traditional Document Management Systems (DMS)
Traditional Document Management Systems (DMS) focus primarily on the storage and organization of documents. DMS platforms allow businesses to digitize paper documents, store them in an electronic format, and retrieve them as needed. This helps reduce reliance on physical storage and enables easy access to files. However, traditional DMS largely operates as document repositories without the capability to intelligently interpret or extract data from the files.
Intelligent Document Processing (IDP)
IDP automates document processing by scanning, reading, and classifying documents with little human intervention. IDP processes document information (supporting several file types) and extracts data intelligently using AI and Natural Language Processing (NLP). Unlike DMS, which merely stores the documents, IDP systems automatically analyze the content and make it searchable and usable within business processes.
Data Extraction and Recognition
Optical Character Recognition (OCR)
OCR is one of the most widely used traditional tools in document processing. It’s designed to read text, including handwritten notes, from scanned documents, converting it into machine-readable text. While OCR marked a major step in digitizing documents, it lacks context or meaning behind the information.
Intelligent Document Processing (IDP)
IDP builds on OCR by integrating machine learning (ML) and natural language processing (NLP). While OCR can extract text, IDP goes a step further to understand the context of that text, identifying relationships between data points and classifying certain document information.
For instance, in an insurance claim document, IDP may distinguish between a policyholder’s name, coverage details, and accident description based on its understanding of the document's structure.
Contextual Understanding and Classification
Traditional DMS and Manual Classification
In traditional systems, documents must be manually categorized by users into folders or indexed using metadata (such as tags or keywords). This is time-consuming and prone to human error. If a document is mislabeled or misplaced, it can be difficult to find later. Traditional DMS offers no automated way of understanding the contents of a document.
Intelligent Document Processing (IDP)
IDP utilizes NLP and AI-powered algorithms to automatically analyze the content of documents and assign them to the correct categories without human input. For example, it can recognize that a document is a contract, an invoice, or a medical record based on the language and structure used. It also identifies key information like names, dates, or specific clauses, making it easier to retrieve relevant data without manual tagging.
Workflow Automation and Decision-Making
Traditional Document Processing
Traditional DMS systems are generally passive, storing documents while leaving decision-making and workflow processes to the user. Any business decisions based on the content of these documents must be made by human workers who manually review the documents, extract necessary data, and input it into other systems.
Intelligent Document Processing (IDP)
IDP transforms documents into actionable data that can seamlessly feed into automated workflows. Using machine learning, IDP systems can automatically route documents to the appropriate department, trigger notifications, and even follow predefined rules. For example, in a claims processing scenario, IDP can extract relevant policy details, assess coverage, and initiate the next steps in the workflow, such as approving a claim or flagging it for further review.
Scalability and Adaptability
Traditional Document Processing
Traditional systems have limitations when it comes to scalability, especially with large volumes of documents. The more documents a business manages, the more challenging it becomes to maintain efficiency, especially since human oversight is still necessary for organizing, tagging, and processing information.
Intelligent Document Processing (IDP)
IDP is designed for scalability. Whether handling thousands or millions of documents, IDP can process large volumes of data efficiently and consistently. Its machine-learning models may continue to improve as they process more documents, becoming increasingly accurate over time. This adaptability makes IDP an ideal solution for growing enterprises, enabling them to manage document-heavy workflows without proportional increases in manpower.
Applications of IDP in Enterprise Companies
Enterprises often deal with a high volume of documents across departments—contracts in legal, invoices in finance, claims in insurance, and compliance documentation in regulatory departments. IDP can transform these workflows in several ways:
Automating Back-Office Processes
Large enterprises sometimes have teams dedicated to manual data entry and document management. IDP can automate these repetitive, error-prone tasks, freeing up employee resources to focus on higher-value activities. For example, IDP can automatically process hundreds or thousands of invoices each day in finance departments, freeing up resources for financial analysis and strategy.
Improving Compliance and Risk Management
Document-intensive industries such as insurance, healthcare, and legal must comply with strict regulatory requirements. Manually reviewing documents for compliance is time-consuming and prone to error. With IDP, companies can automate compliance checks, flag potential risks, and ensure that clauses in contracts or policies are correctly identified. This is particularly important in industries where small deviations can lead to significant legal or financial repercussions.
Enhancing the Customer Experience
For enterprise companies in sectors like commercial insurance, speed is paramount to customer satisfaction. IDP allows companies to process claims far more quickly than manual methods. By automating document review and decision-making processes, enterprises can offer a faster, more seamless customer experience.
Boosting Data-Driven Decision Making
Many enterprise decisions are based on the analysis of document-driven data. However, extracting this data from complex documents can be challenging. IDP enables enterprises to extract valuable data at scale, transforming it into formats that can feed into analytics platforms or business intelligence tools. This enables faster, more accurate decision-making based on real-time insights.
Limitations of IDP
Struggles with Unstructured Long-Form Documents
IDP excels in processing structured or semi-structured documents such as forms, invoices, or purchase orders, where fields are predefined and data is organized consistently. However, IDP often falls short when dealing with unstructured or long-form documents like contracts, insurance policies, and legal agreements. These documents often contain complex narratives, various formats, and highly variable structures that IDP systems struggle to process accurately.
Extensive IT Setup and Maintenance
Many IDP systems require significant IT infrastructure and ongoing support. They often need custom models and rules, which involve large investments in terms of time and technical expertise. This complexity can delay implementation and create a dependency on technical teams for setup, maintenance, updates, or new functionality.
Predefined Templates and Rigid Models
Traditional IDP systems often rely on predefined templates and rules to extract data. These systems work well when document formats are consistent, but they can break down when faced with highly variable documents that don't adhere to strict structures. As a result, they may miss important information that doesn’t fit their predefined model.
Lack of Contextual Understanding
While IDP can extract specific data points like names, dates, or amounts, it can often hit limits in the ability to detect contextual relationships. For instance, distinguishing between dates or other details in a renewal clause versus a liability clause in a legal document may require a deeper comprehension of the document's content, something many IDP systems aren’t inherently designed to do.
How to Scale Across Enterprises with Document Engineering
While IDP focuses on extracting and processing data from semi-structured or structured documents, Document Engineering—a more advanced methodology—takes it a step further by automatically handling unstructured, long-form, complex business documents and turning them into structured data that can be fully utilized by enterprises, without painstaking IT setup.
Cloud-Based Solutions for Scalability
Cloud-based Document Engineering platforms, such as those offered by Docugami, enable enterprises to process documents at scale, without the need for extensive internal infrastructure. The cloud allows for scalability, making it easy for businesses to ramp up document processing when demand increases. Additionally, cloud-based solutions ensure that the Document Engineering software can integrate with other cloud-based systems like ERP platforms.
Customizable and Trainable Models
One of the most significant advantages of Document Engineering is its ability to be trained on specific document types and workflows. Enterprises can customize these models to meet their unique needs, whether it’s processing specific types of contracts or regulatory documents. Machine learning models can continuously improve as they process more documents, becoming more accurate over time.
AI-Augmented Human Review
Although IDP automates much of the document processing work, certain use cases—such as complex legal contracts—may still require human judgment. Document Engineering systems leave room for human review when needed, providing a balance between automation and human oversight. This combination of AI and human intelligence ensures accuracy, especially in highly regulated industries like insurance and law.
Compliance and Security
When processing sensitive documents at scale, enterprises must ensure that their IDP solution adheres to strict data security and compliance standards. Document Engineering providers like Docugami ensure that data is processed securely, meeting regulations such as GDPR and SOC 2 compliance.
Real-World Example: Transforming Document Processing with Docugami
Docugami, a leader in document engineering and AI-powered document solutions, exemplifies the potential of IDP for enterprise companies. By focusing on transforming complex business documents into structured, reusable data, Docugami helps businesses automate processes and fundamentally rethink how they use documents to drive business value. Its platform enables users to create new document workflows, extract precise information, and integrate that data into business systems—all without needing extensive technical expertise.
For example, in the commercial insurance industry, Docugami’s IDP solution allows brokers and underwriters to automatically extract key policy details, clauses, and exclusions from lengthy insurance documents, speeding up workflows, improving accuracy and highlighting necessary data insights. Document content becomes the knowledge graph for decisions that it was meant to be, without reading pages and pages of narrative and tabular text.
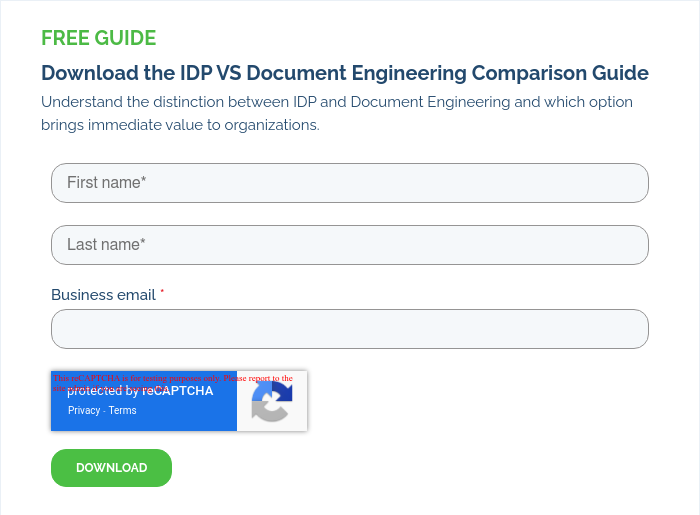
Conclusion
Intelligent Document Processing is an important technology that empowers enterprises to extract more value from their documents. By automating the ingestion, classification, and understanding of unstructured data, IDP has enabled companies to scale their operations, improve efficiency, and make better decisions based on real-time data.
That said, while IDP offers significant benefits for automating document processing, it is often limited when dealing with complex, unstructured documents. In contrast, Document Engineering goes still further to offer a more advanced, flexible solution that can handle the intricacies of long-form documents, deliver contextual insights, and require minimal technical overhead. By moving beyond predefined, programmed data extraction, Document Engineering enables businesses to unlock deeper value from their document data.
For enterprise companies looking to streamline processes, reduce costs, and stay competitive in the digital age, Document Engineering offers a clear path forward. As leading providers like Docugami continue to innovate in this space, the future of document processing and document intelligence will be faster, smarter, and more transformative than ever before.